Machine Learning Magic: How Google Search Incorporates AI to Enhance Results
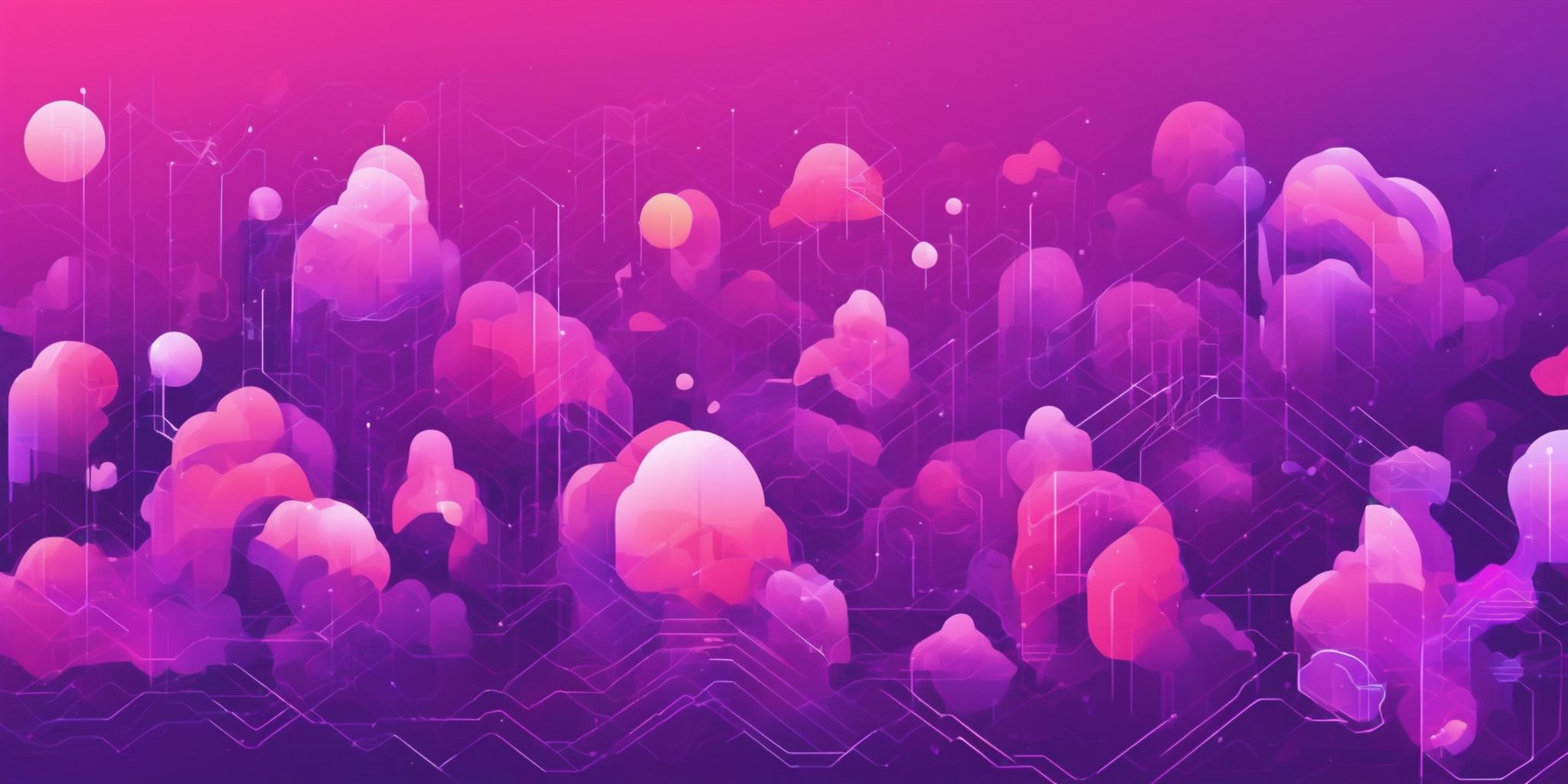
Picture this: it's a lazy Sunday afternoon, and you decide to embark on a daring quest—to find the perfect chocolate chip cookie recipe. Armed with your trusty laptop, you turn to the holy grail of information: Google Search. As you start typing, anticipating a list of mouthwatering options, little do you know that behind the scenes, an enchanting force known as machine learning is quietly at work.
Join us as we unveil the secret magic behind Google's search engine, revealing how artificial intelligence (AI) wields its powers to deliver more relevant and personalized results than ever before. Get ready to be spellbound by the tantalizing wonders of machine learning, as we dive into the realm where algorithms and data seamlessly blend with human curiosity and imagination.
Evolution of Google Search
The early days of Google's search algorithm
In the early days, Google's search algorithm relied on basic rules and algorithms to rank web pages. Keywords played a significant role in determining search results. However, this approach had limitations in providing relevant and accurate information to users. With the integration of machine learning, Google's search algorithm evolved to better understand user intent and context.
By analyzing patterns and data from billions of web pages, machine learning algorithms can now provide more accurate search results, even understanding nuances and synonyms. This technology continues to improve, ensuring users receive the most relevant information for their queries.
Understanding Machine Learning
Definition and explanation of machine learning
Machine learning is a subset of artificial intelligence that enables computers to learn and improve from experience without being explicitly programmed. In the context of Google Search, machine learning algorithms analyze vast amounts of data to better understand user intent and provide more relevant search results. These algorithms can identify patterns, detect relationships, and make predictions based on historical data.
For example, when a user searches for "best camera for photography," machine learning algorithms can analyze past search queries, user behavior, and content relevance to deliver the most helpful results. By continuously learning and adapting, machine learning enhances the accuracy and effectiveness of Google Search.
Benefits of using machine learning in search algorithms
One of the benefits of incorporating machine learning in search algorithms is improved relevance. Machine learning allows search engines like Google to analyze vast amounts of data and understand semantics, context, and user intent. By learning from user behavior and preferences, search algorithms can better predict what users are looking for and provide more accurate results.
For example, if a user often clicks on search results related to a specific topic, the algorithm can prioritize similar results in the future. This leads to a more personalized and tailored search experience for users, increasing satisfaction and engagement.
Additionally, machine learning helps to identify patterns and trends in user queries, enabling search engines to anticipate and offer relevant suggestions or autocomplete options.
Google's Integration of AI in Search
How Google uses machine learning to improve search results
Google uses machine learning to improve search results by constantly analyzing vast amounts of data and patterns. This enables the search engine to understand user intent and provide more relevant and accurate results. Machine learning algorithms take into account various factors such as user location, search history, and context to deliver personalized and contextual results.
For example, if a user frequently searches for recipes, the algorithm may prioritize recipe websites in their search results. This helps refine the overall search experience and ensure that users find what they are looking for more efficiently.
Examples of machine learning in Google Search
Examples of machine learning in Google Search can be seen through features like autocomplete suggestions and spell correction. Through machine learning algorithms, Google's search engine analyzes user behavior and data patterns to predict and provide relevant search suggestions. This helps users save time and find information faster.
Additionally, machine learning aids in improving spell correction by understanding common misspellings and offering corrected suggestions. These examples demonstrate how machine learning enhances the search experience by personalizing results and making search queries more efficient and accurate.
RankBrain: Google's Machine Learning Algorithm
How RankBrain improves search relevance
RankBrain, a key component of Google's machine learning algorithm, enhances search relevance by understanding and interpreting search queries more effectively. It has the ability to analyze complex search terms and connect them with relevant content, even if the exact words or phrases used in the query aren't present on a webpage.
For example, if someone searches for "best Italian restaurant near me," RankBrain can identify that the user is looking for recommendations on local Italian restaurants. By considering various factors like location, user behavior, and context, it delivers more accurate and tailored search results. This improves user satisfaction and ensures that the search engine understands the intent behind the search query.
RankBrain's impact on SEO practices
RankBrain, Google's machine learning algorithm, has significantly influenced the landscape of SEO practices. Its impact can be observed in the following ways:
- Improved understanding of search intent: RankBrain's ability to interpret complex queries has made search results more relevant and personalized.
- Emphasis on user experience: Websites that prioritize user satisfaction by providing valuable content and seamless navigation tend to rank higher.
- Semantic search optimization: Traditional keyword optimization has given way to a focus on context and related concepts to enhance search visibility.
- Quality content triumphs: High-quality, informative content that addresses user queries in depth has gained importance over keyword stuffing.
- Enhanced user engagement: Engaging elements such as videos, images, and interactive features contribute to better user engagement, positively impacting SEO rankings.
Personalized Search Results using Machine Learning
Understanding personalized search
Understanding personalized search is essential in the context of Google search machine learning. It refers to the customization of search results based on individual user preferences, search history, and behavior patterns. By analyzing user data and employing machine learning algorithms, Google can deliver more relevant and tailored search results to each user.
For example, if a user frequently searches for fitness-related topics, Google may prioritize fitness-related websites in their search results. This personalization enhances the user experience by saving time and providing more accurate information. However, it is important to note that personalized search also raises concerns about privacy and filter bubbles, where users may be exposed to a limited range of viewpoints.
Machine learning techniques for personalization
Machine learning techniques for personalization help Google Search deliver tailored results to individual users. By analyzing user behavior and preferences, Google can understand their intent and provide more relevant content. This is achieved through various methods, including collaborative filtering, content-based filtering, and reinforcement learning.
Collaborative filtering recommends items based on similarities between users, while content-based filtering considers the characteristics of the items themselves. Reinforcement learning improves personalization by optimizing search rankings based on user feedback.
For example, if a user frequently clicks on articles related to "healthy recipes," Google will prioritize showing similar content in future searches. These techniques enhance user experience by delivering targeted information and improving user satisfaction.
Benefits and implications of personalized search results
Personalized search results offer several benefits to users.
Firstly, they enhance user experience by presenting more relevant content based on individual preferences and past behavior. This saves time and effort, as users can quickly find what they are looking for.
Secondly, personalized results can improve the accuracy of search predictions, aiding in anticipating user needs.
For example, if a user frequently searches for vegan recipes, the search engine can prioritize showing vegan-related content. However, there are implications to consider as well. Personalized results may create filter bubbles, limiting exposure to diverse viewpoints. Moreover, privacy concerns arise as user data is used to tailor search results. It is essential to strike a balance to ensure personalized search benefits users while addressing potential disadvantages.
Future of Google Search with Machine Learning
Advancements and improvements expected in the future
Advancements and improvements in machine learning will continue to shape the future of Google Search. As algorithms become more sophisticated, search results will become even more relevant and personalized to individual users. This means that users can expect to find information that aligns better with their specific needs and preferences.
Additionally, natural language processing capabilities will improve, allowing users to have more conversational interactions with search engines. With advancements in image and voice recognition, users will also be able to search using visual or voice inputs, enhancing the overall search experience. These developments will revolutionize the way we search and retrieve information, making it more intuitive and efficient.
Implications for users and content creators
- Users will experience more relevant and personalized search results, increasing user satisfaction and efficiency.
- Content creators need to understand and adapt to the evolving algorithms by optimizing their content for user intent rather than just keyword stuffing.
- Creating high-quality, valuable content becomes even more crucial as machine learning algorithms prioritize quality over quantity.
- It is essential for content creators to analyze and understand user behavior, preferences, and search patterns to tailor their content accordingly.
- Content creators should focus on user engagement metrics like click-through rates, time on page, and bounce rates to gauge the effectiveness of their content and make necessary adjustments.
Conclusion
Google Search is leveraging the power of artificial intelligence and machine learning to improve its search results. The incorporation of AI allows Google to understand and interpret search queries more effectively, providing users with more accurate and relevant information. Machine learning algorithms not only help in understanding the context, but also in identifying patterns and trends among search queries.
This enables Google to offer personalized suggestions and predictions based on individual preferences and past searches. By utilizing AI, Google Search aims to continually enhance the user experience and deliver more tailored and comprehensive search results.