The future of content recommendation algorithms with AI
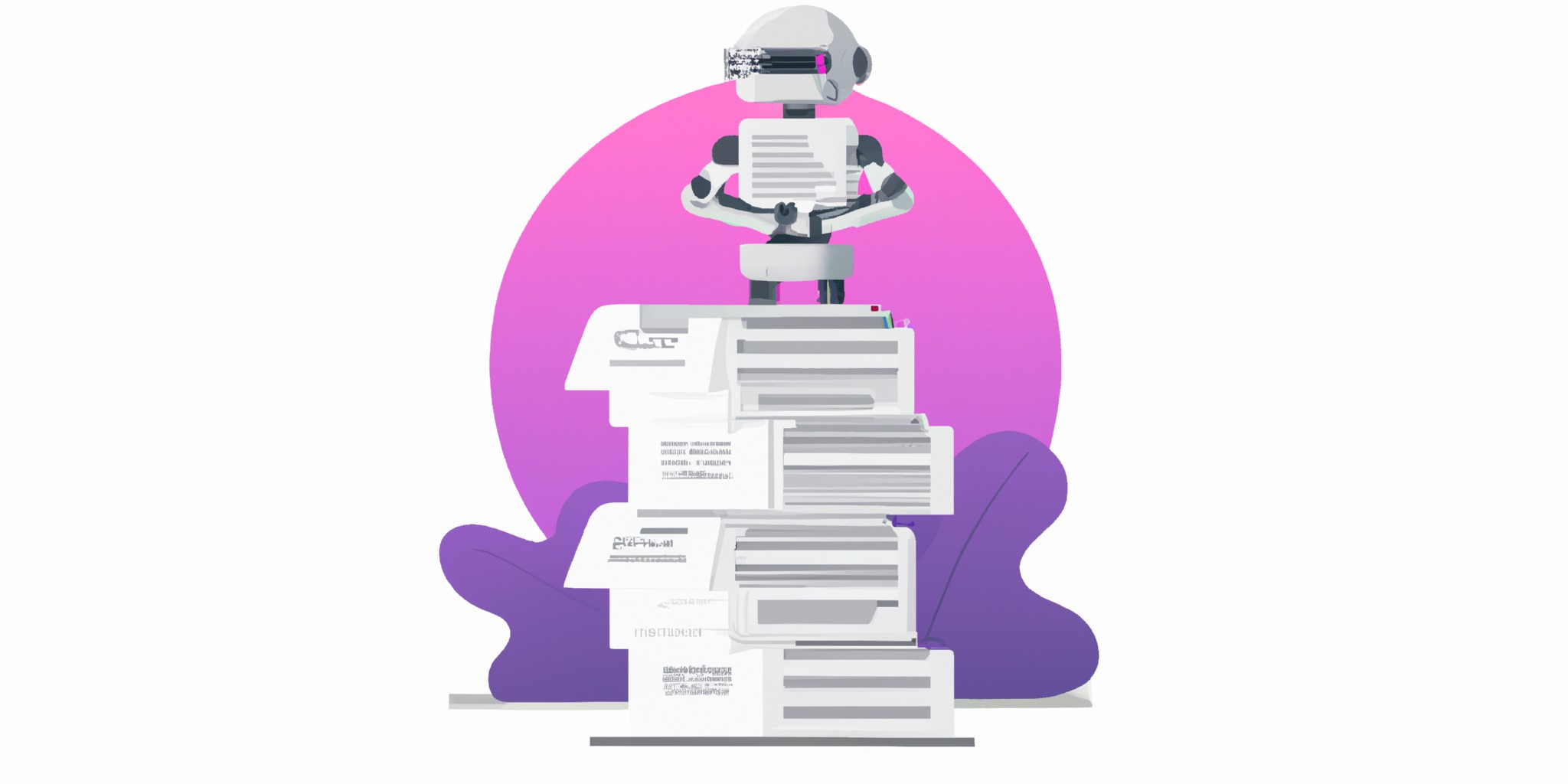
We're all familiar with the experience of scrolling endlessly through social media feeds, streaming services, and news websites, desperately searching for something to catch our eye. It can be overwhelming, to say the least. But what if we told you that in the not-so-distant future, this frustrating experience could be a thing of the past? With the rapid development of artificial intelligence (AI), content recommendation algorithms are becoming smarter, more efficient, and more personalized than ever before. In fact, these algorithms are poised to revolutionize the way we consume content, making it easier for us to discover and enjoy the things that truly matter to us. So what does the future of content recommendation algorithms look like, and how will AI help shape it? Let's take a closer look.
The evolution of content recommendation algorithms
The evolution of content recommendation algorithms has been a fascinating journey, and it all started with the simple concept of suggesting similar content based on user behavior. However, over time, content recommendation algorithms have become more sophisticated and personalized. Initially, the recommendations were based on data such as viewing history, search queries, and user preferences. But now, with the help of AI, content recommendation algorithms can analyze vast amounts of data to create highly personalized and contextually relevant recommendations.
In recent years, there has been a shift towards using more complex machine learning algorithms, such as deep learning, to improve the accuracy of recommendations. These algorithms are trained to recognize patterns and relationships in data, which allows them to make more intelligent suggestions based on a user's interests and behavior.
Furthermore, the evolution of content recommendation algorithms has been driven by the need to make recommendations across different platforms and devices. As users consume content across a range of devices, such as smartphones, tablets, and smart TVs, recommendation algorithms must be able to adapt and provide a consistent experience across all platforms.
In conclusion, the evolution of content recommendation algorithms has been a continuous process, driven by technological advancements and the need to provide users with a more personalized and seamless experience. With AI and machine learning leading the way, it's exciting to think about the potential for these algorithms to continue evolving and improving in the future.
The impact of AI on content recommendation algorithms
AI has had a significant impact on content recommendation algorithms, revolutionizing the way we discover and consume content. Traditional recommendation algorithms were limited to analyzing a user's past behavior to provide suggestions, but AI has enabled recommendation algorithms to take a more proactive approach, analyzing a user's behavior and preferences in real-time to make personalized recommendations.
One of the biggest impacts of AI on content recommendation algorithms is the ability to use natural language processing (NLP) to understand the context and sentiment of content. This allows algorithms to make more nuanced and personalized recommendations based on the content's topic, tone, and emotional impact.
AI has also enabled recommendation algorithms to incorporate more data points beyond just a user's past behavior. For instance, it can analyze a user's social media activity, location, and other contextual factors to make recommendations that are more relevant to their current situation.
Moreover, AI has helped address the issue of filter bubbles, which occurs when users are only exposed to content that aligns with their existing beliefs and biases. By analyzing a user's behavior and preferences, AI can provide recommendations that challenge their existing views, exposing them to diverse and different perspectives.
In conclusion, the impact of AI on content recommendation algorithms has been profound, making recommendations more relevant, personalized, and diverse. The possibilities are endless, and we can expect AI-powered recommendation algorithms to continue improving and shaping the way we consume content in the years to come.
The role of user data in personalized recommendations
The role of user data is critical to personalized recommendations as it allows recommendation algorithms to make more accurate and relevant suggestions based on a user's behavior, interests, and preferences. User data is collected through various channels, such as website activity, search queries, social media interactions, and purchase history.
Personalized recommendations rely heavily on user data, as algorithms use this information to analyze patterns and trends in a user's behavior to make tailored suggestions. For example, if a user consistently watches videos on a particular topic, the algorithm will take note of this behavior and provide more similar content.
One of the main advantages of using user data for personalized recommendations is that it enables algorithms to make suggestions based on a user's current interests and preferences, rather than just their past behavior. This real-time analysis allows recommendation algorithms to adapt quickly to a user's changing needs and interests.
However, there are also potential ethical concerns around the collection and use of user data, such as data privacy and security. Companies must be transparent about their data collection practices and provide users with the ability to opt-out or delete their data if they choose to do so.
In conclusion, the role of user data in personalized recommendations is essential, as it allows algorithms to provide more relevant and accurate suggestions based on a user's behavior and preferences. However, it's also important for companies to be transparent about their data practices and ensure that users' privacy and security are protected.
The ethical implications of content recommendation algorithms
While content recommendation algorithms have undoubtedly improved the way we discover and consume content, there are also significant ethical implications associated with their use. One of the main concerns is the potential for recommendation algorithms to create "filter bubbles" where users are only exposed to content that confirms their existing beliefs and biases.
This can lead to a lack of exposure to different perspectives, contributing to a polarized society. Furthermore, recommendation algorithms can perpetuate stereotypes and reinforce discrimination by recommending content based on factors such as race, gender, and ethnicity.
There are also concerns around data privacy and security, as recommendation algorithms rely on collecting and analyzing user data to make personalized suggestions. Companies must be transparent about their data collection practices and ensure that users' privacy and security are protected.
Another ethical consideration is the potential for recommendation algorithms to manipulate user behavior by promoting certain content over others. This can have far-reaching consequences, such as influencing elections, perpetuating fake news, and promoting harmful content.
To address these ethical concerns, companies need to be transparent about their recommendation algorithms and how they are making suggestions to users. They should also consider the use of human curation alongside AI to ensure that recommendations are not solely based on data analysis.
In conclusion, while content recommendation algorithms have many benefits, there are also significant ethical considerations associated with their use. Companies need to be proactive in addressing these concerns to ensure that recommendation algorithms are used responsibly and do not contribute to societal harms.
How content recommendation algorithms will change the way we consume media
Content recommendation algorithms are already changing the way we consume media, and their impact is only going to become more significant in the future. These algorithms use AI and machine learning to analyze user data and make personalized suggestions based on a user's interests, behavior, and preferences.
One of the most significant changes that content recommendation algorithms will bring is a more personalized media experience. Instead of browsing through endless options, users will receive tailored recommendations that match their interests and preferences. This will save time and effort, making it easier for users to discover new content that they enjoy.
Another significant change is the potential for content recommendation algorithms to break down traditional barriers between different types of media. For example, users who enjoy reading about a particular topic may be recommended podcasts, videos, or documentaries on the same subject, expanding their media consumption beyond their usual formats.
Furthermore, recommendation algorithms can help to promote diversity and inclusivity in media consumption. By analyzing user data and recommending content that challenges existing beliefs and biases, algorithms can expose users to a broader range of perspectives and opinions, promoting greater understanding and empathy.
Overall, content recommendation algorithms will revolutionize the way we consume media by making it more personalized, diverse, and convenient. While there are potential ethical concerns associated with their use, companies can take steps to ensure that recommendation algorithms are used responsibly and do not contribute to societal harms.
The potential for AI-powered content discovery in niche areas
The potential for AI-powered content discovery in niche areas is immense, as recommendation algorithms can help users discover content in areas that are traditionally underserved. Niche areas can include anything from specialized hobbies and interests to scientific research and academic fields.
AI-powered recommendation algorithms can be particularly useful in niche areas where users may have difficulty finding content that matches their interests. By analyzing user data and recommending relevant content, algorithms can help users discover new topics and areas of interest that they may not have found on their own.
One of the most significant advantages of AI-powered content discovery in niche areas is the potential for greater diversity and inclusivity. Traditional media channels may not always serve niche audiences effectively, but AI-powered recommendation algorithms can help to surface content that is both relevant and diverse. This can help to promote greater understanding and appreciation for different cultures, perspectives, and interests.
Furthermore, AI-powered recommendation algorithms can help to support emerging creators and content providers in niche areas. By recommending content to users who may not have found it otherwise, algorithms can help to build a broader audience for niche content and support the growth of new creators and providers.
Overall, the potential for AI-powered content discovery in niche areas is significant, as it can help users discover new topics and areas of interest while promoting greater diversity and inclusivity in media consumption. As these algorithms continue to evolve, we can expect to see even greater opportunities for content discovery in previously underserved areas.
The future of content recommendation algorithms in e-commerce and advertising
The future of content recommendation algorithms in e-commerce and advertising is promising, as these algorithms can help businesses to deliver more personalized and targeted content to their customers. By analyzing customer data and behavior, recommendation algorithms can make personalized suggestions that are more likely to lead to sales and conversions.
In e-commerce, recommendation algorithms can help users to find products that match their interests and preferences, increasing the likelihood of a purchase. For example, if a user has shown an interest in a particular type of product, a recommendation algorithm can suggest similar products that they may be interested in purchasing.
In advertising, recommendation algorithms can help businesses to target their ads more effectively to users who are more likely to be interested in their products or services. By analyzing user data, algorithms can determine which users are more likely to make a purchase and deliver ads to those users, increasing the efficiency of advertising campaigns.
Furthermore, as recommendation algorithms become more sophisticated, we can expect to see even greater opportunities for businesses to use them to drive sales and conversions. For example, AI-powered recommendation algorithms could help businesses to predict which products a user is likely to purchase in the future, allowing them to make more targeted recommendations and offers.
However, there are potential ethical concerns associated with the use of recommendation algorithms in e-commerce and advertising. Businesses must ensure that they are transparent about their data collection practices and that user privacy and security are protected. They should also consider the potential for algorithmic bias and take steps to mitigate any harmful effects.
Overall, the future of content recommendation algorithms in e-commerce and advertising is bright, as they offer significant opportunities for businesses to deliver more personalized and targeted content to their customers. As these algorithms continue to evolve, we can expect to see even greater potential for driving sales and conversions while also addressing ethical concerns associated with their use.
The limitations and challenges of AI-powered content recommendation algorithms
While AI-powered content recommendation algorithms have the potential to transform the way we consume media and discover new content, there are several limitations and challenges associated with their use.
One of the main limitations of these algorithms is the potential for algorithmic bias. If recommendation algorithms are not designed and trained carefully, they can perpetuate existing biases and discrimination, resulting in recommendations that are not inclusive or representative of diverse perspectives. Additionally, if algorithms are not regularly updated and tested, they can quickly become outdated and less effective.
Another challenge associated with these algorithms is the issue of data privacy and security. Recommendation algorithms rely on vast amounts of user data to function effectively, which can raise concerns about how that data is collected, stored, and used. To address these concerns, businesses and developers must ensure that they are transparent about their data collection practices and implement strong data security measures.
Another limitation of AI-powered content recommendation algorithms is the potential for oversimplification. While these algorithms are designed to make personalized recommendations based on user data, they may not always take into account more nuanced or complex factors that influence user preferences and behavior. For example, an algorithm may recommend a certain type of content based on a user's search history, but it may not take into account other contextual factors, such as the user's mood or location.
Finally, there is the challenge of striking a balance between personalization and serendipity. While users may appreciate personalized recommendations that align with their interests, they may also want to discover new and unexpected content that they may not have found on their own. Striking this balance requires careful consideration of how recommendation algorithms are designed and implemented.
Overall, while AI-powered content recommendation algorithms offer significant opportunities for improving the way we discover and consume media, there are also several limitations and challenges that must be addressed. By being mindful of these challenges and taking steps to address them, we can continue to improve the effectiveness and inclusivity of these algorithms and ensure that they are used in ways that benefit users and society as a whole.
The role of human curation in the age of AI-powered recommendations
As AI-powered content recommendation algorithms become more prevalent, there is still an important role for human curation in the age of digital media. While algorithms can help to make personalized recommendations based on user data, human curators bring a level of creativity, diversity, and expertise that algorithms cannot replicate.
Human curation can help to ensure that recommendations are more diverse and inclusive, representing a wide range of perspectives and content types. This is especially important in areas such as news and journalism, where algorithms may be prone to perpetuating biases and misinformation.
Additionally, human curation can bring a level of creativity and personal touch to content recommendations that algorithms may not be able to replicate. For example, a human curator may be able to identify connections between seemingly unrelated topics or recommend content based on their personal expertise and experience.
Moreover, human curators can also help to ensure that content recommendations are ethical and aligned with the values of their organization or community. This includes ensuring that content is factually accurate, respectful, and appropriate for the intended audience.
However, human curation also has its limitations. It is more time-consuming and resource-intensive than automated algorithms, and it may not be scalable for large volumes of content. Additionally, human curators may be prone to their own biases and subjectivities, and there is a risk of gatekeeping and censorship if curators are not transparent and accountable in their decision-making.
Overall, the role of human curation in the age of AI-powered recommendations is an important one. By combining the strengths of both human curation and automated algorithms, we can ensure that content recommendations are more diverse, inclusive, creative, and ethical, while also being scalable and efficient.
The future of content recommendation algorithms and the changing landscape of media consumption
As content recommendation algorithms become more sophisticated and widespread, they are poised to revolutionize the way we consume media and discover new content. The future of these algorithms is likely to be shaped by several key trends in the changing landscape of media consumption.
One major trend is the shift towards mobile and on-demand media consumption. As more people consume media on their mobile devices and expect content to be available on-demand, recommendation algorithms will need to adapt to these changing user behaviors. This may involve optimizing for shorter attention spans and developing more targeted and personalized recommendations for mobile users.
Another trend is the increasing importance of social media and user-generated content in the media landscape. As more people turn to social media platforms for news and entertainment, recommendation algorithms will need to be able to incorporate social signals and user-generated content into their recommendations. This may involve developing more sophisticated algorithms that can analyze user interactions and behaviors across multiple platforms.
Additionally, the growing demand for personalized and niche content is likely to shape the future of content recommendation algorithms. As more people seek out content that aligns with their specific interests and preferences, recommendation algorithms will need to be able to provide more personalized and targeted recommendations. This may involve incorporating more diverse data sources and developing more advanced machine learning models.
Moreover, the growing awareness of ethical issues surrounding content recommendation algorithms is likely to influence their future development. As concerns around algorithmic bias and data privacy continue to grow, developers and businesses will need to be more transparent and accountable in the way they design and implement these algorithms.
Overall, the future of content recommendation algorithms is likely to be shaped by a combination of technological advancements, changing user behaviors, and ethical considerations. By staying abreast of these trends and adapting to the evolving needs and expectations of users, recommendation algorithms can continue to play a vital role in the way we discover and consume media.
Over to you
In recent years, content recommendation algorithms powered by AI have become an increasingly important part of the digital media landscape. These algorithms use data analytics and machine learning to make personalized recommendations to users, helping them to discover new content and stay engaged with digital media.
In this article, we explore the future of content recommendation algorithms with AI and their potential to revolutionize the way we consume media. We cover several key subtopics, including the evolution of these algorithms, the impact of AI on content recommendation, the role of user data in personalized recommendations, the ethical implications of these algorithms, the potential for AI-powered content discovery in niche areas, and the future of these algorithms in e-commerce and advertising.
We also examine the limitations and challenges of AI-powered content recommendation algorithms and the role of human curation in the age of automated recommendations. Finally, we explore the changing landscape of media consumption and the trends that are likely to shape the future of these algorithms.
Overall, this article provides a comprehensive overview of the current state and future potential of content recommendation algorithms with AI. As these algorithms continue to evolve and become more sophisticated, they have the potential to transform the way we discover and consume media, while also raising important ethical and societal questions that will need to be addressed.
Want boost your traffic with AI-generated content? Start for free.